Overview:
In a large-scale retrospective validation involving over 1,700 consecutive patients presenting to German Chest Pain Units, the PMcardio STEMI AI ECG Model demonstrated high diagnostic accuracy (95.3%) and specificity (96.7%) in identifying high-risk patients typically missed by standard ECG interpretation. This highlights its potential to significantly improve emergency care through earlier detection and intervention in a real-world, broad clinical setting.
Presented at: German Society for Cardiology (DGK) 90th Annual Congress
Presented Date: April 10, 2024
Background
Occlusion myocardial infarction (OMI) might be missed by conventional ST-elevation myocardial infarction (STEMI) criteria on 12-lead surface electrocardiogram (ECG). Twenty-five percent of non-STEMI patients have OMI in worldwide registries. These patients are at risk for delayed invasive management resulting in poor short- and long-term prognosis.
Artificial intelligence (AI) model studies have shown robust performance in detecting angiographically determined OMI using individual 12-lead surface ECGs. However, these studies were conducted in high-risk patient populations with a high prevalence of OMI and more data is needed to evaluate the utility of AI in a broader chest pain (CP) population.
We investigated the performance of an AI ECG model detecting angiographic OMI in a German metropolitan chest pain unit (CPU) cohort of all-comer patients without typical STEMI criteria from a tertiary care center.
Methods
The AI model was developed and validated in international cohorts including >12,000 CP patients with an OMI prevalence of around 20%. The present study included all consecutive patients presenting to CPU who were either ruled out by serial high-sensitive cardiac troponin T (hs-cTnT) testing or referred for angiography after CPU assessment.
STEMI patients diagnosed in the prehospital phase were excluded from the analysis (Figure 1). In Germany, peak troponin is not routinely measured. Consequently, an angiographic OMI outcome was modeled including identification of culprit lesion, coronary artery flow and hs-cTnT.
The AI model performance was assessed according to sensitivity, specificity, accuracy and predictive values in the overall cohort and in the subgroup of ruled-out population following 0/1h hs-cTnT algorithm. Receiver operating characteristic (ROC) analyses were performed.
Results
In total, 1776 ECGs from 1770 CPU patients were considered. The mean age of patients was 53.2 (±18.9) years, and 62.1% were male. Eighty percent of patients were ruled-out by serial hs-cTnT measurement, and the remaining 354 patients were referred for invasive management.
OMI prevalence was 3.16%. In the overall analysis, the AI ECG model achieved an area under the curve of 0.894 in identifying OMI (Figure 2 and table 1). Sensitivity was 0.518 (95% CI: 0.386-0.649), and specificity reached 0.967 (95% CI: 0.959-0.976). Correspondingly, negative predictive value (NPV) was 98.4% (95% CI: 97.8-99.0), and positive predictive value (PPV) was 34.1% (24.2-44.4). Diagnostic accuracy was 0.953 (95% CI: 0.943-0.963).
Subgroup analysis of patients ruled-out following 0/1h hs-cTnT algorithm identified misclassification as false-positive in 1% of ECGs by AI model. Specificity and accuracy both were 0.989 (95% CI: 0.983-0.994, see table 2). Negative predictive value was 100% (95% CI: 100-100).
Conclusion
This external validation study of a pre-existing in AI ECG model demonstrates remarkable diagnostic accuracy in a German CPU cohort without a pre-clinical diagnosis of STEMI. The AI model was reliable with high specificity and resulted in overcalls only in few cases.
Sensitivity analysis of the AI ECG model was restricted by the low prevalence of OMI and by the modeled OMI definition given the German CPU specifics. The present study proved technical feasibility, but future studies are required to further evaluate its applicability in German CPUs.
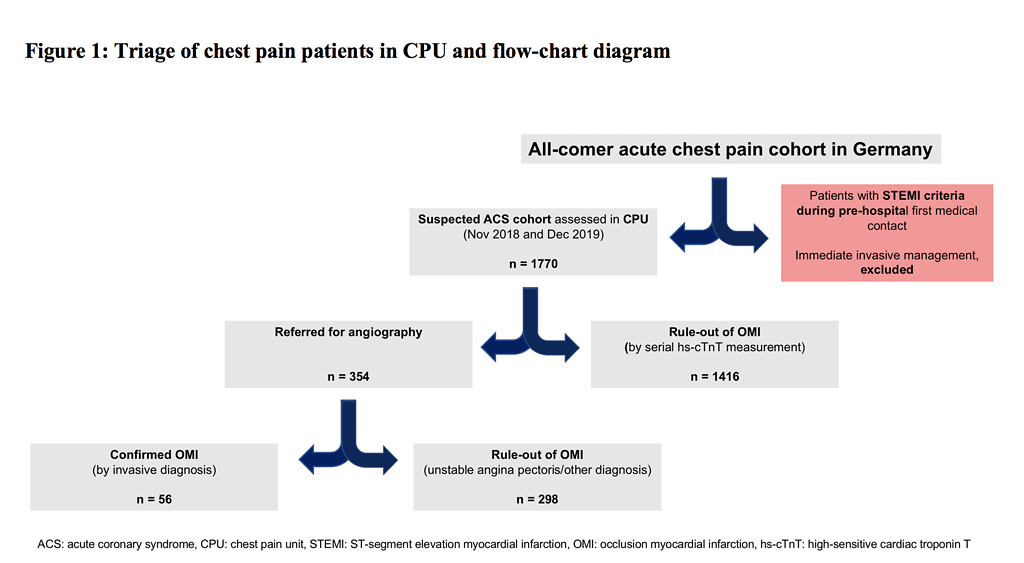
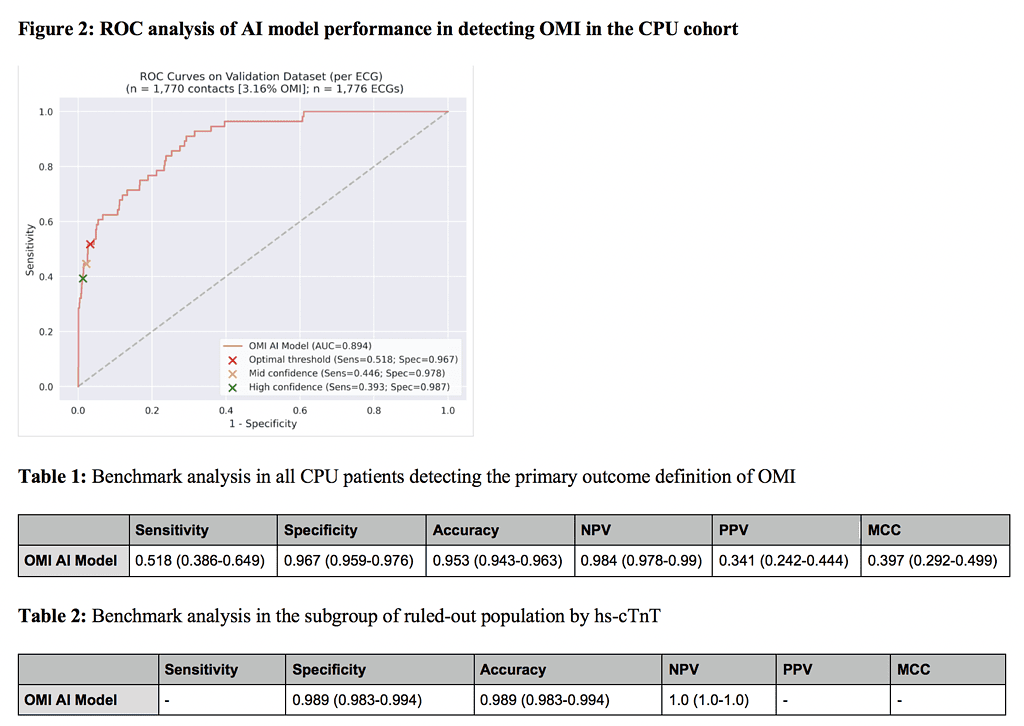
Authors: Sascha Macherey-Meyer (Köln), P. Meyers (Charlotte, NC), S. Smith (Minneapolis), F. Hundehege (Köln), L. Krücken (Köln), S. Heyne (Köln), M. Meertens (Mainz), S. Braumann (Köln), V. Mauri (Köln), S. Baldus (Köln), S. Lee (Köln), C. Adler (Köln)