An electrocardiogram (ECG) is a vital diagnostic tool used to evaluate the functioning of the cardiovascular system. By detecting the electrical signals and heartbeat of the patient, the ECG is used to diagnose and treat various cardiac disorders. Its widespread use has made it an essential tool in modern medicine.
In the past decades, automated ECG interpretation has been widely used. Some levels of automation of ECG interpretation have been around since the 1970s. Until this day, however, computer-aided ECG interpretation remains relatively inaccurate, as multiple studies have shown.
There is already more advanced technology based on machine learning algorithms and deep neural networks that provide AI-powered ECG interpretation that is as accurate as that of an expert cardiologist with 30 years of experience.
In this article, we’ll dive deeper into the latest use of AI in cardiology and explore how simple AI ECG interpretation app based on deep learning technology revolutionizes how healthcare professionals work with ECGs.
AI in ECG:Table of Contents
Our CMO, Robert Herman, MD, discusses the future roadmap for leveraging AI models in ECG interpretation on the Mayo Clinic podcast.
How does the automated ECG interpretation work?
Early attempts to automate ECG interpretation (still widely used in many interpretation algorithms – typically built into standard ECG machines) were based on algorithms that are programmed using pattern recognition and conditional statements (if-then-else) that tell a program to execute an action depending on whether a condition is true or false.
This technology relies on the detection of fiducial points in an ECG signal.
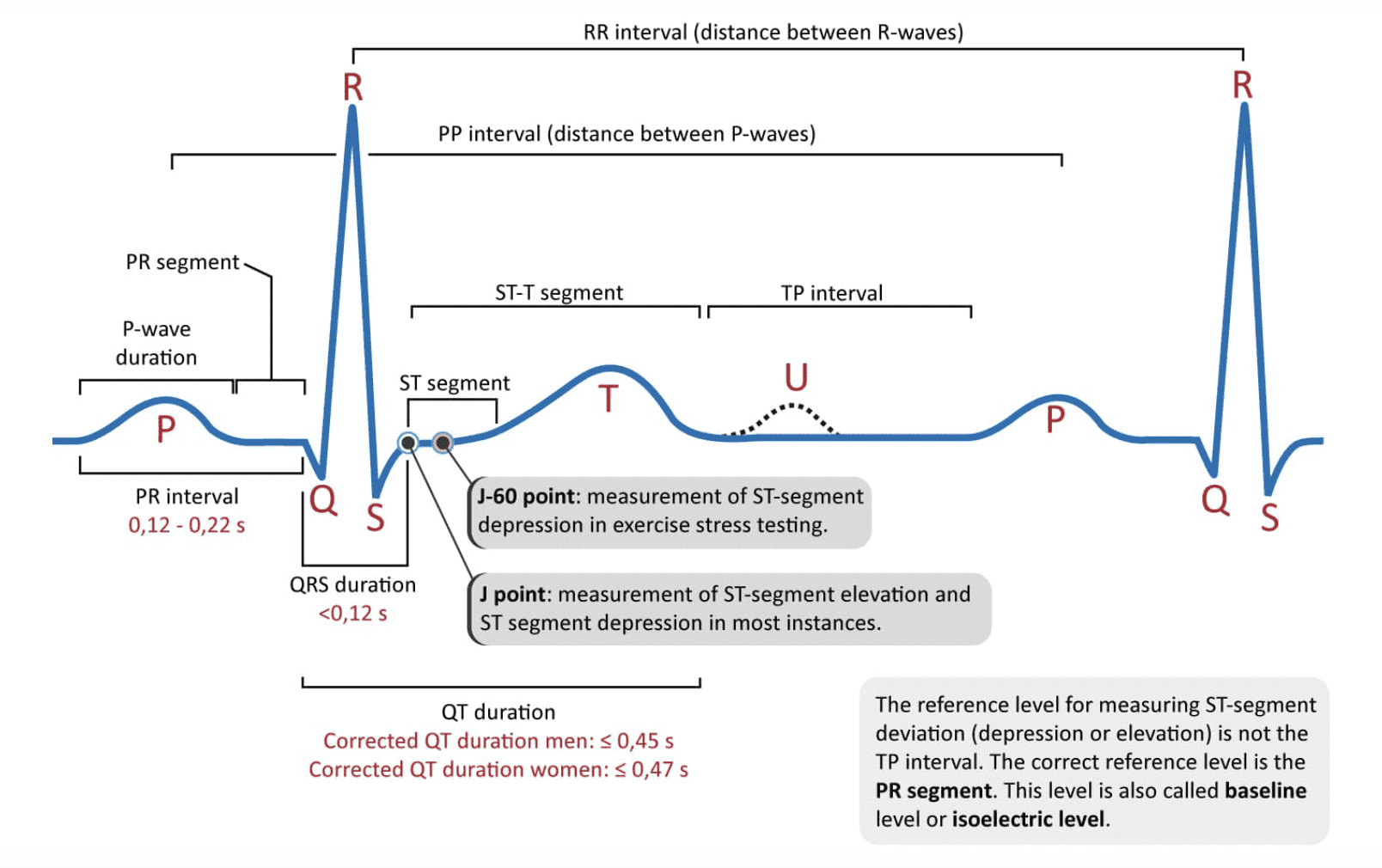
Using these and a set of expert-defined rules, the algorithms classified various cardiac conditions. For example, a simplified algorithm to detect Atrial Fibrillation from an ECG would look as follows:
- Detect main fiducial points on an ECG
- Compute the variance in the R-R interval a. Does it pass a certain threshold?
- Detect atrial activity – P waves a. No regular atrial activity detected? b. Are High-frequency fibrillatory waves present?
This technology, however, has many limitations.
What are some challenges with automated ECG interpretation as we know it today?
The current automated ECG interpretation technology has multiple challenges and limitations that may significantly affect the algorithm’s accuracy.
1. There is a lack of standardization in electrocardiography
There’s a limitation regarding which diagnoses can be ‘formalized’ into a decision tree using an almost universal set of rules, which does not capture the nuance and complexity inherent to many conditions. ECG interpretation is still subjective, and there needs to be more consensus on some cardiac diagnoses, for example, Left Bundle Branch Block criteria or the diagnosis of LVH from an ECG. Furthermore, the formalization of diagnoses into if-else conditions could lead to the introduction of biases against specific patient cohorts.
2. Traditional computerized ECG solutions can be sensitive to noise interference
The existing algorithms rely heavily on accurately detecting often subtle fiducial points on the ECG, which is challenging, especially in the presence of noise or minor noise artifacts. These occur quite often, e.g. high-frequency noise that renders a waveform fuzzy or makes the baseline of the waveform wander. These can trick the interpretation algorithm and lead to misinterpretation.
3. ECG interpretation is highly dependent on the patient’s clinical parameters
Besides the ECG waveform itself, parameters like age and gender, but mainly the patient’s symptoms, have a massive influence not only on the resulting diagnosis but also on the triage and subsequent management of the patient. Current automated ECG interpretation algorithms lack the ability to contextualize these findings with the broader clinical context and fail to provide guideline-adherent treatment recommendations.
AI ECG interpretation is the future: How does machine learning-powered ECG diagnostic work?
ECG is all about pattern recognition, and machines are good at it – why not leave the pattern recognition part up to the computer?
Read your next ECG with AI
Be part of a future where cardiac care is smarter, faster, and more accessible. Sign up now and claim your 5 free ECG reports – no credit card needed.
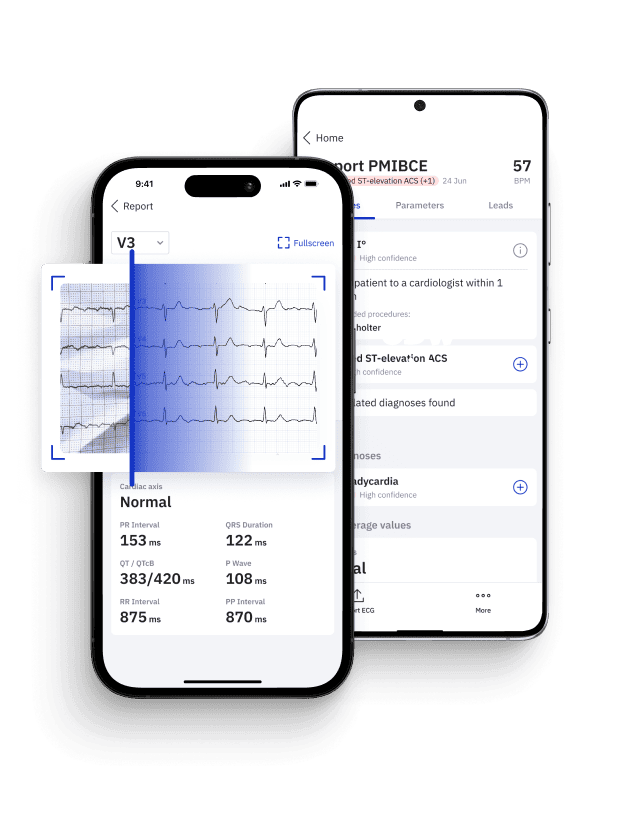
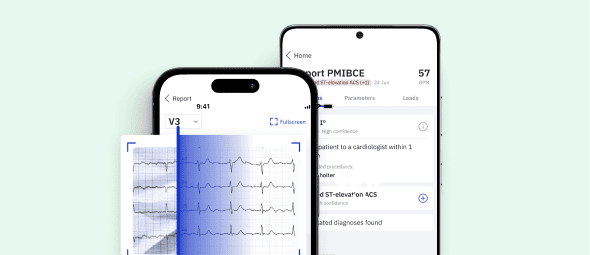
Just imagine – what if we could show the computer previous ECG examples and the respective patient outcomes and let the computer find and associate the patterns that lead to a certain outcome? In other words, let the computer teach itself and master ECG interpretation.
Let’s look at how this machine learning-powered ECG interpretation works – a complex task requiring substantial expertise.
While the automated ECG interpretation technology found in standard ECG machines (described above) is based on classical programming (pattern recognition and conditional rules), the future of ECG interpretation lies in advanced machine-learning technology based on neural networks that are able to learn from the data and create its own rules for interpreting ECGs. No patterns are directly given as input; any patterns are learned in the process of training.
Here’s the visual representation of the difference between the two technologies.
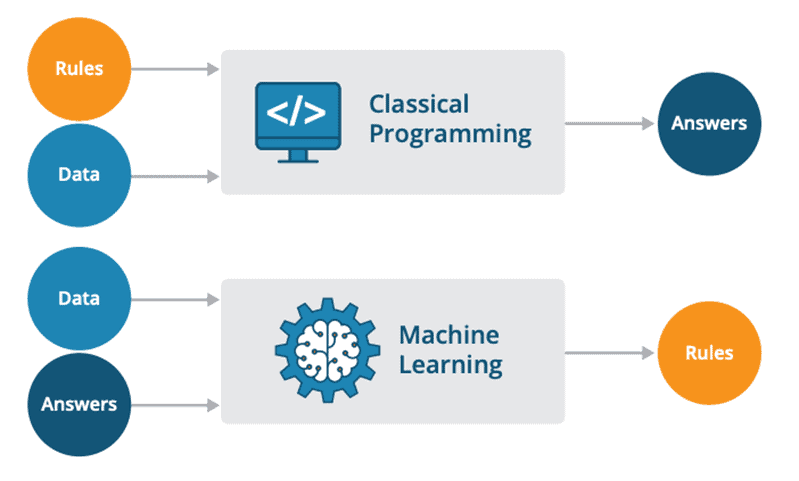
This is where AI (and PMcardio) joins the ECG interpretation game – by training AI algorithms on ECGs with patient outcomes; the AI can detect specific patterns and associate them with a certain outcome (diagnoses, measurements, etc.).
By crunching millions of previous ECGs in a matter of hours, the machine can spot and learn even very subtle patterns possibly missed by the human eye.
To illustrate this on a practical example, PMcardio’s deep neural network (deep learning algorithm) model has been trained on a total of 931,344 standard 12-lead ECGs from 172,750 patients to detect 38 individual diagnoses of heart rhythms, heart blocks, infarctions, hypertrophies, and ectopies among others.
The diagnostic performance of PMcardio has been validated on the largest globally representative cohort of patients. In a head-to-head benchmark, PMcardio has outperformed primary care physicians and achieved non-inferior performance to cardiologists across all evaluated diagnoses, demonstrating statistically significant diagnostic superiority.
Moreover, PMcardio goes beyond mere diagnosis. After generating an ECG report, it prompts diagnosis-specific clinical questions for accurate patient context. By merging ECG diagnostics with these parameters, our engine provides tailored patient management advice, detailing specific treatments and drugs while adhering to the latest ESC guidelines.
Case study: AI-powered ECG interpretation technology in the detection of STEMI
As we already mentioned above, the accuracy of automatic electrocardiogram interpretation can vary depending on the program and the ECGs’ over-readers and the patient’s parameters, such as age, sex, and race.
For example, in the detection of ST-segment elevation myocardial infarction (STEMI), the automatic electrocardiogram interpretation technology shows quite a high inaccuracy. In a study published in 2022, a team of experts found out that the diagnostic accuracy of auto-interpretation was 73.5%, whereas 26.5% were misinterpreted as non-acute myocardial infarctions.
In numbers, the automated ECG interpretation technology misinterpreted ST-segment elevation myocardial infarction in 31 out of 117 patients (26.5%).
In detecting ST-elevation myocardial infarctions, PMcardio was tested on 3,820 ECG test cases in a clinical trial. The PMcardio app demonstrated 31% better diagnostic accuracy when compared to cardiologists and also reduced falsely negative results by 97%, showing significant diagnostic superiority.
PMcardio showed superior performance in detecting STEMI infarctions, with a higher PPV (0.90) and Sensitivity (0.99) compared to cardiologists (0.95 and 0.76, respectively), while NPV and Specificity were similar (1.00 and 0.92 for PMcardio 2.6, 0.85 and 0.97 for cardiologists).
The figure below shows a 31% increase, from 123 to 161 incorrectly interpreted STEMI infarctions by PMcardio 2.6 compared to cardiologists. For NSTEMI infarctions, PMcardio 2.6 had higher PPV (0.95) and Sensitivity (0.83) compared to cardiologists (0.85 and 0.87, respectively), with similar NPV (0.94 for PMcardio 2.6, 0.95 for cardiologists) and Specificity (0.98 for both).
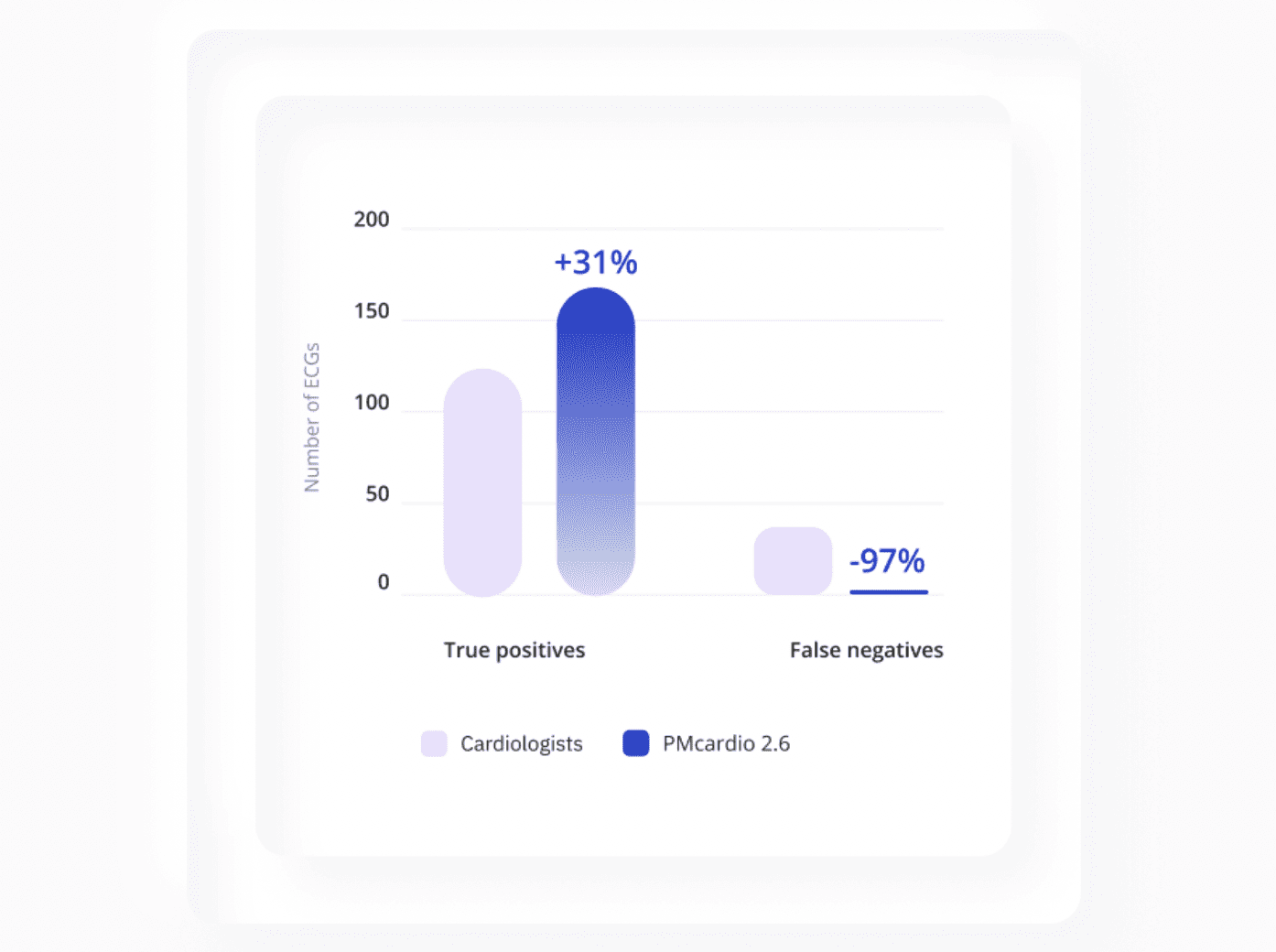
Conclusion
In conclusion, AI and machine learning are transforming the field of ECG interpretation by providing faster and more accurate diagnoses of cardiovascular diseases. With the use of deep neural networks, patterns and abnormalities in ECG waveforms can be recognized more effectively, allowing for early detection and prediction of future diseases.
This new advanced technology not only reduces the workload for doctors but also saves lives by enabling healthcare professionals to make informed decisions quickly and accurately. As AI continues to become a vital part of healthcare, the potential to ensure more accurate diagnosis and reduce the number of medical errors is becoming a reality.
Stay on the pulse with our newsletter
Get access to the latest news on AI progress within emergency hospital care.
Your submission was successful
FAQ: AI ECG interpretation
What is the AI on the ECG?
Artificial intelligence (AI) applied to electrocardiogram (ECG) interpretation employs advanced machine learning and deep learning techniques. This AI-driven approach excels in pattern recognition, rapidly identifying subtle cardiac abnormalities from vast datasets of ECG records. Unlike traditional methods that depend on static rules, AI algorithms continually learn and adapt, improving their diagnostic accuracy over time.
Is there an app that interprets ECG?
Yes, PMcardio is an app designed and certified to assist healthcare professionals by interpreting electrocardiograms (ECGs) using advanced artificial intelligence technology. This tool directly enhances the reliability of cardiac assessments, providing detailed diagnostics and treatment recommendations based on official guidelines.
How does AI-powered ECG interpretation work?
AI utilizes machine learning and deep neural networks to interpret ECGs. Unlike traditional methods that rely on predefined rules and pattern recognition, AI systems learn from large datasets of previous ECGs, identifying patterns and correlating them with outcomes. This allows AI to detect subtle abnormalities that might be missed by human eyes.
Can ChatGPT read an ECG?
While ChatGPT-4 Plus has shown some capability in interpreting ECG images in test settings, it is not a reliable tool for clinical ECG analysis. The interpretation of ECGs demands precise, expert evaluation, and AI chatbots like ChatGPT do not meet the necessary standards for medical diagnostics. Always consider using specialized AI solutions like PMcardio, designed a certified to deliver expert-level ECG diagnostics with high accuracy and speed.
What app reads ECG?
PMcardio is an AI ECG interpretation app that reads ECGs. It quickly and accurately diagnoses up to 39 cardiovascular conditions from any 12-lead ECG. Certified for use by healthcare professionals, PMcardio enhances cardiac care by providing reliable diagnostics and treatment recommendations within seconds.